HarVI: Real-Time Intervention Planning for Coronary Artery Disease Using Machine Learning
International Conference on Computational Science
Cyrus Tanade and Amanda Randles
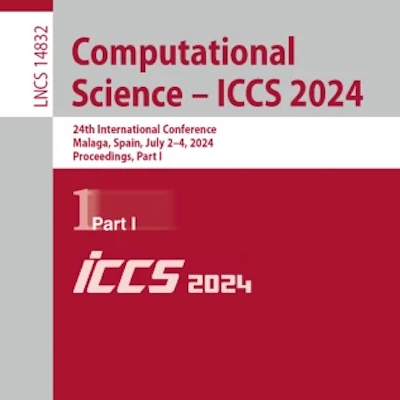
Summary
Virtual planning tools that provide intuitive user interaction and immediate hemodynamic feedback are crucial for cardiologists to effectively treat coronary artery disease. Current FDA-approved tools for coronary intervention planning require days of preliminary processing and rely on conventional 2D displays for hemodynamic evaluation. Immersion offered by extended reality (XR) has been found to benefit intervention planning over traditional 2D displays. To bridge these gaps, we introduce HarVI, a coronary intervention planner that leverages machine learning for real-time hemodynamic analysis and extended reality for intuitive 3D user interaction. The framework uses a predefined set of 1D computational fluid dynamics (CFD) simulations to perform one-shot training for our machine learning-based blood flow model. In a cohort of 50 patients, we calculated fractional flow reserve (FFR), the gold standard biomarker of ischemia in coronary disease, using HarVI (FFRHarVI) and 1D CFD models (FFR1D). HarVI was shown to almost perfectly recapitulate the results of 1D CFD simulations through continuous and categorical validation scores. In this study, we establish a machine learning-based process for virtual coronary treatment planning with an average turnaround time of just 74 min, thus reducing the required time for one-shot training to less than a working day.
Citation
Tanade, Cyrus, and Amanda Randles. “HarVI: Real-time intervention planning for coronary artery disease using machine learning.” International Conference on Computational Science. Cham: Springer Nature Switzerland, 2024.